Over the past five years, the number of robotics startups has surged. We’ve seen videos of robots moving materials, cooking, cleaning, sorting, and even dancing. This proliferation naturally leads to a modern Fermi paradox: “If there is so much activity, where are all the robots in real life?” In Ati’s context, factories remain relatively devoid of robots, with human-operated vehicles dominating material movement.
Traditionally, the slow adoption of robots was attributed to high costs. However, sensor prices have dropped significantly in recent years, making robots more affordable than ever. Additionally, advancements in algorithms, the software ecosystem, and the availability of talent have all improved. So, what is holding back widespread adoption?
A subtler reason, particularly in industrial settings, is the fear of new technology. As consumers, we are quick to try robotic vacuum cleaners or robot chefs based on online reviews. However, line managers worry about production backlogs if a robot fails. Safety managers are concerned about the implications of robots working in hybrid environments, and IT chiefs fear introducing cybersecurity threats. For robotics firms, proving that robots can operate safely, efficiently, and reliably in customer workflows is crucial. Achieving safety standard certifications and security compliances can be arduous, but these steps are necessary to gain the trust of stakeholders. Then there is the question of operational reliability.
The first successful use case for robots was the Roomba, which was a stroke of genius. Even if it initially vacuumed only half of your house properly, it still saved you work. However, finding another use case where a robot can be useful without being perfect has been challenging, especially in industrial environments. Operations teams demand high reliability, often in the high 90s, before integrating robots into their processes. Startups, which traditionally ship early and improve iteratively with customers, struggle with this demand for near-perfection from the outset. Achieving incremental reliability improvements from 90% to 95% to 99% is not linear; it requires solving a long tail of edge cases and achieving robustness. There is hope that an all-encompassing neural network might one day address this.
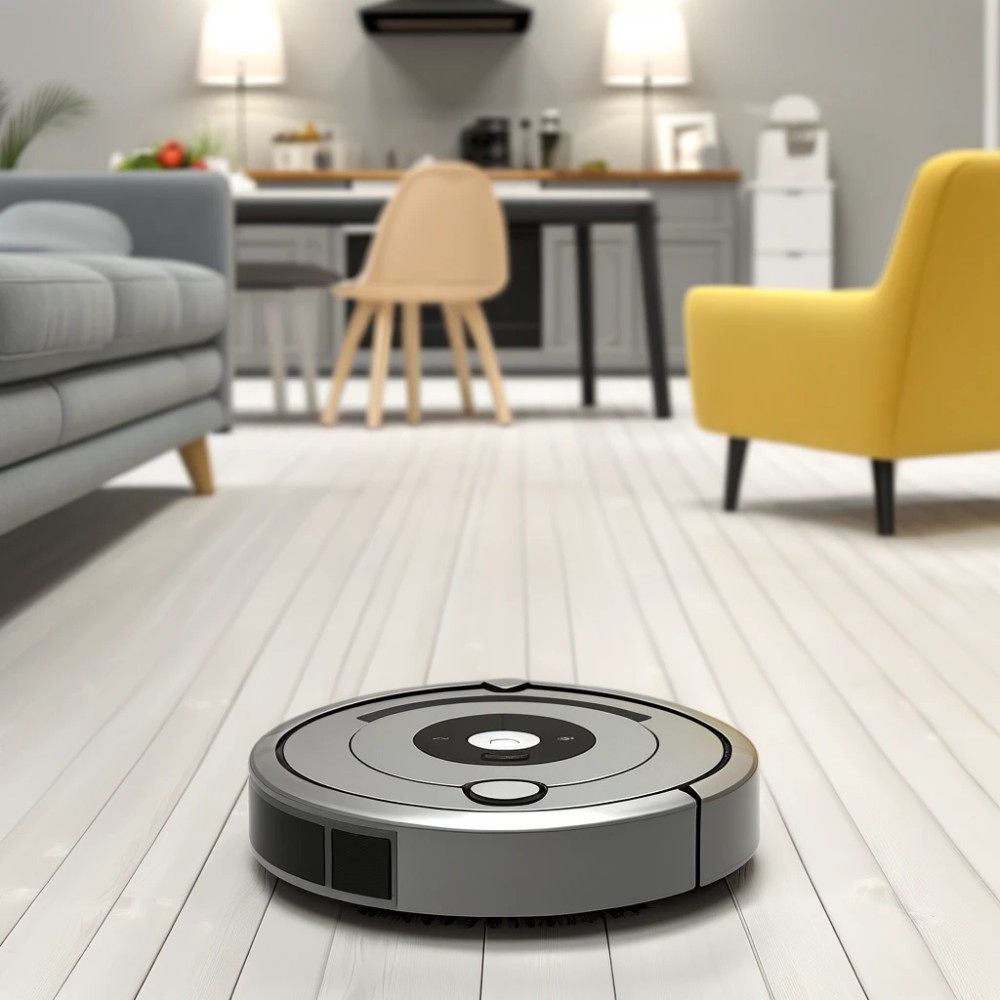
Recently, at a high school running event in the US, I was cheering for my family. The 100m school record was listed at 10.8 seconds, just one second shy of Usain Bolt’s record! But at competitive level fractional speed improvements require months of dedicated training, attention to nutrition, and mental fortitude. Similarly, while initial success in robotics comes relatively quickly, achieving world-class operational stability in production environments takes sustained effort. Tens of thousands of virtual and real iterations are necessary. Ati’s home-grown simulator has played an important role in weeding out edge cases before robots go live. Round-the-clock manual testing provides assurance of endurance and repeatability. With 100+ robots in the field we are learning everyday at Ati.
Reflecting on Jensen Huang’s recent proclamation at GTC’ 24: “Everything that moves will become robotic at some point.” To reach this future, the robotics industry must conquer the long tail of edge cases.